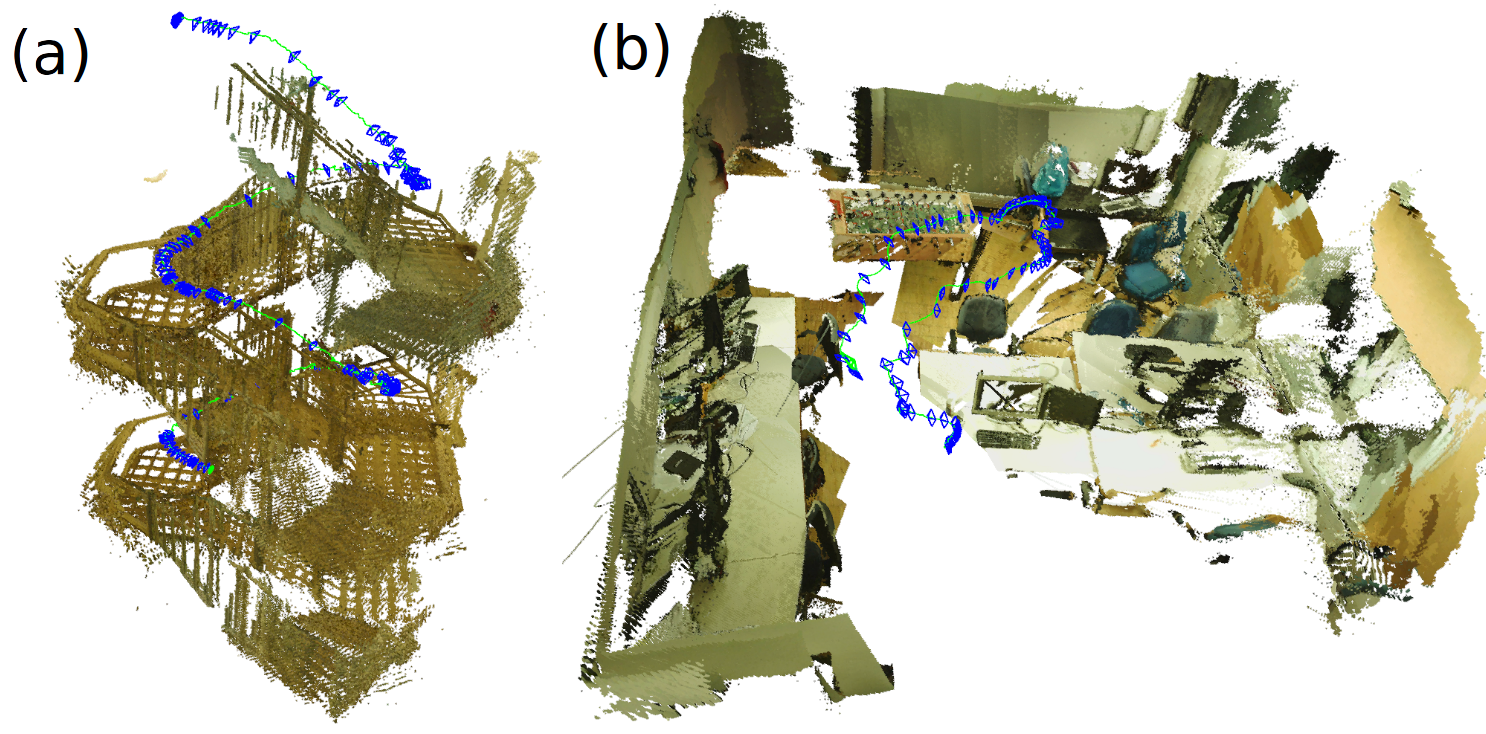
In this work, we present a robust edge-based visual odometry (REVO) system for RGBD sensors. Edges are more stable under varying lighting conditions than raw intensity values, which leads to higher accuracy and robustness in scenes, where feature- or photoconsistency-based approaches often fail. The results show that our method performs best in terms of trajectory accuracy for most of the sequences indicating that edges are suitable for a multitude of scenes.
Our system runs in real-time on the CPU of a laptop computer.
Code is available: REVO at github
If you use our framework or parts of it, please city any amount of the following publications:
- Combining Edge Images and Depth Maps for Robust Visual Odometry
Schenk, F., Fraundorfer, F.
British Machine Vision Conference (BMVC) 2017 [pdf] [video] - Robust Edge-based Visual Odometry using Machine-Learned Edges
Schenk, F., Fraundorfer, F.
2017 IEEE/RSJ International Conference on Intelligent Robots and Systems (IROS) 2017 [pdf] [video]