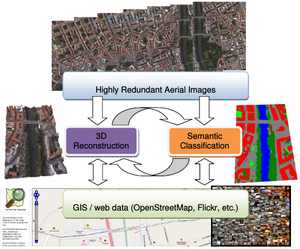
The aim of this research project is holistic scene understanding in large aerial datasets, consisting of thousands of massively redundant high-resolution images. Holistic scene understanding is one of the major problems in computer vision and photogrammetry and has recently got a lot of attention. The problem of holistic image understanding includes two fundamental tasks: 3D scene reconstruction and semantic interpretation of the imaged content at the level of pixels. The tight interaction between semantic classification and 3D reconstruction is often ignored by state of the art aerial image processing workflows, due to the lack of computational power, the absence of efficient algorithms or the enormous effort of manual intervention. However, these tasks are mutually informative and should be solved jointly as a correct class labelling is a valuable source of information for reconstruction, and 3D information can help to improve the semantic interpretation. For instance, a correct classification is a valuable source of information for reconstruction in regions where dense matching methods fail (e.g. sheets of water and reflecting windows / facades), and 3D information can be used as a prior to improve classification (e.g. building and road detection). The high resolution and redundancy due to large overlaps of aerial images requires massive processing power which will be handled by taking advantage of graphic processing units that have proved to give a significant speedup compared to single core machines. In particular, we will focus on algorithms based on variational methods, which provide a high degree of parallelization capability. In order to reduce cost-intensive manual interaction, we further will exploit publicly available user-data from the Internet to improve both interpretation and 3D reconstruction.
In the HOLISTIC project we will provide a flexible framework for scene classification and 3D reconstruction from aerial images that outperforms current state-of-the art and delivers interpretable models at highest possible accuracy. To achieve this goal, we will focus our attention on the following two research subjects: (i) the joint optimization of geometry and semantic classification from aerial images in a unified framework, and (ii) the exploitation of existing geographic information systems and web data to support these two sub-tasks. In addition, we will use web-based standard to efficiently represent the obtained results for fast modeling and data parsing.